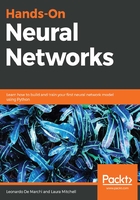
Environment setup
There are only a few viable programming language options when creating ML software. The most popular ones are Python and R, but Scala is also quite popular. There are other languages, but the better ones in terms of use in ML are Julia, JavaScript, Java, and a few others. In this book, we will be using Python only. The motivation behind this choice is its widespread adoption, its simplicity of use, and the vast ecosystem of libraries that are possible to use.
In particular, we will be using Python 3.7 and a few of its following libraries:
- numpy: For fast vectorized numerical computation
- scipy: Built on top of numpy, with many mathematical functionalities
- pandas: For data manipulation
- scikit-learn: The main Python library for ML
- tensorflow: The engine that powers our deep learning algorithms
- keras: The library we are going to use to develop our deep learning algorithms, which sits on top of TensorFlow
Let's focus on the last two libraries for the moment. There are a few libraries that are useful nowadays for Neural Networks (NNs). The most widespread is TensorFlow. It is a symbolic math library that uses a directed graph to model the dataflow between operations.
It's particularly suitable for matrix multiplications as it can use all the power of the GPU's architecture, composed by many, but not particularly powerful, cores that can execute many operations simultaneously. TensorFlow is also quite versatile as it works on a few different platforms; it's possible to run models on mobile devices using TensorFlow Lite and even on a browser using TensorFlow.js (https://www.tensorflow.org/js).
One of the libraries we are going to use for most of this book will be Keras. It is a layer that sits on the top of libraries such as TensorFlow to provide a more abstract interface to the end users. In this regard, Keras is narrower than TensorFlow as it focuses on neural networks, but it's also more generic as it can be used in conjunction with TensorFlow's alternatives such as the Microsoft Cognitive Toolkit.
Now, these libraries don't guarantee backward compatibility. Because of this, when working with Python, it's good practice to work in virtual environments. This allows us to isolate the different projects we are working on, but also to distribute our setup to different machines in an easy way and to avoid compatibility issues.
There are a few ways we can create these environments; in this book, we will cover the following:
- Virtual environment
- Anaconda
- Docker
We will go through each one of them to show how they work and explain their advantages and disadvantages.