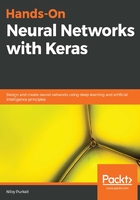
Learning through errors
All we essentially do to our input data is compute a dot product, add a bias term, pass it through a non-linear equation, and then compare our prediction to the real output value, taking a step in the direction of the actual output. This is the general architecture of an artificial neuron. You will soon see how this structure, configured repetitively, gives rise to some of the more complex neural networks around.
Exactly how we converge to ideal parametric values by taking a step in the right direction is through a method known as the backward propagation of errors, or backpropagation for short. But to propagate errors backwards, we need a metric to assess how well we are doing with respect to our goal. We define this metric as a loss, and calculate it using a loss function. This function attempts to incorporate the residual difference between what our model thinks it sees, and the actual ground reality. Mathematically speaking, this is shown as (y - ). It is important to understand here that the loss values can actually be defined as a function of our model parameters. Thus, tweaking these parameters permits us to reduce our loss and get our predictions closer to actual output values. You will see exactly what we mean by this when we review the full training process of our perceptron.