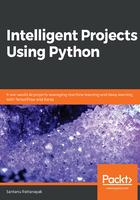
Long short-term memory (LSTM) cells
The vanishing gradient problem is taken care of, to a great extent, by a modified version of RNNs, called long short-term memory (LSTM) cells. The architectural diagram of a long short-term memory cell is as follows:

LSTM introduces the cell state, Ct, in addition to the memory state, ht, that you already saw when learning about RNNs. The cell state is regulated by three gates: the forget gate, the update gate, and the output gate. The forget gate determines how much information to retain from the previous cell states, Ct-1, and its output is expressed as follows:

The output of the update gate is expressed as follows:

The potential new candidate cell state, , is expressed as follows:

Based on the previous cell state and the current potential cell state, the updated cell state output is given via the following:

Not all of the information of the cell state is passed on to the next step, and how much of the cell state should be released to the next step is determined by the output gate. The output of the output gate is given via the following:

Based on the current cell state and the output gate, the updated memory state passed on to the next step is given via the following:

Now comes the big question: How does LSTM avoid the vanishing gradient problem? The equivalent of in LSTM is given by
, which can be expressed in a product form as follows:

Now, the recurrence in the cell state units is given by the following:

From this, we get the following:
As a result, the gradient expression, , becomes the following:

As you can see, if we can keep the forget cell state near one, the gradient will flow almost unattenuated, and the LSTM will not suffer from the vanishing gradient problem.
Most of the text-processing applications that we will look at in this book will use the LSTM version of RNNs.