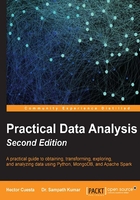
Data reduction methods
Many data scientists use large data size in volume for analysis, which takes a long time, though it is very difficult to analyze the data sometimes. In data analytics applications, if you use a large amount of data, it may produce redundant results. In order to overcome such difficulties, we can use data reduction methods.
Data reduction is the transformation of numerical or alphabetical digital information derived empirically or experimentally into a corrected, ordered, and simplified form. Reduced data size is very small in volume and comparatively original, hence, the storage efficiency will increase and at the same time we can minimize the data handling costs and will minimize the analysis time also.
We can use several types of data reduction methods, which are listed as follows:
- Filtering and sampling
- Binned algorithm
- Dimensionality reduction
Filtering and sampling
In data reduction methods, filtering plays an important role. Filtering explains the process of detecting and correcting errors from raw data. After getting the filtered data, we can use them as input data for succeeding analysis. The filters look like mathematical formulas. There are many filtering methods available for extracting errors and noise-free data from raw data. Some of the filter methods are moving average filtering, Savitzky-Golay filtering, high correlation filtering, Bayesian filtering, and many more. These filters should be adopted in a proper manner based on raw data and the context of the study.
Most of the filters are applied to a sample of raw data. For example, in Bayesian filtering methods, we can use a sample of data, that comes based on the Monte Carlo sequential sampling method.
For data reduction using filtering, the sampling techniques play an important role. The importance of sampling is to extract statistical inferences about the population from sample data. Large data stored in the databases is normally called "population" data. In the data reduction process, we extract a subset of data that best represents the population.
Binned algorithm
Binning is a classification process for extracting a small set of groups or bins from a continuous variable. Binning is widely used in many fields like genomics and credit scoring. More frequently, binning is used at an early stage to select variables from the specified fields. To enhance the predictive power, similar attributes of an independent variable are grouped into the same bin.
Commonly used binning algorithms are:
- Equal-width binning: Values are divided into a predefined number of bins of equal width intervals.
- Equal-size binning: Attributes are sorted first and then divided into a pre-defined number of equal-size bins.
- Optima binning: Data divided into a large number of initial equal-width bins, say 20. These bins are then treated as categories of a nominal variable and grouped to the required number of segments in a tree structure.
- Multi-interval discretization binning: This binning process is the minimization of entropy for binary discretizing the range of a continuous variable into multiple intervals and recursively defining the best bins.
For selecting proper binning algorithm, we should consider the following strategies:
- Missing values are binned separately
- Each bin should contain at least 5% of observations
- No bins have 0 accounts for good or bad
- Weight of Evidence (WOE) is a quantitative method for combining evidence in support of a statistical hypothesis
- Binning algorithms are available in Python 3.4 software also, for example,
import binningx0dt
command
Dimensionality reduction
Dimensionality reduction methods should be applied for taking a fewer sequence of orthogonal latent variables, instead of observed explanatory variables as dependent variables in the predicting process. This means dimensionality reduction is converting very high-dimensionality data into much lower dimensionality data, such that each of the lower dimension data conveys more information.
Dimensionality reduction process is a statistical or mathematical technique, in which we can describe most, but not all, of the variance within our data, but retain the relevant information. In statistics, the process of dimension reduction can reduce the number of random variables and can be divided into feature selection and feature extraction. The following diagram represents the process of data reduction:

There are many techniques available to tackle dimensionality reduction. Principle Component Analysis (PCA) and Linear Discriminant Analysis (LDA) are the most widely used techniques. Comparatively, LDA will give better results than PCA for big datasets.
PCA is a multivariate data analysis technique. Using this technique, we can explain the underlying variance-covariance structure of a large set of variables through a few linear combinations of these variables.
The objective of LDA is to perform dimensionality reduction while preserving as much of the class discriminatory information as possible. LDA finds most discriminant projection by maximizing between-class distance and minimizing within-class distance.