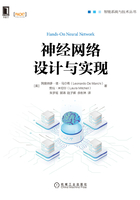
上QQ阅读APP看书,第一时间看更新
2.4.1 FFNN的Keras实现
为了在Keras中实现我们的网络,我们将再次使用Sequential模型,但是因为这次需要进行二分类预测,所以需要一个输入神经元、三个隐藏单元以及一个输出单元。
1)导入创建网络所需的部分:

2)现在,我们需要定义网络的第一个隐藏层。为此,只需指定隐藏层的输入即可(在XOR情况下为两个)。我们还可以指定隐藏层中神经元的数量,如下所示:

3)选择使用tanh作为激活函数:

4)然后,我们添加具有一个神经元的另一个全连接层,该层的激活函数为sigmoid,以此为我们提供输出:

5)再次使用SGD作为优化方法来训练我们的神经网络:

6)然后,编译神经网络,指定使用MSE作为损失函数:

7)作为最后一步,我们训练网络,但是这次我们不在乎批次大小,运行2个epoch:

8)像往常一样,我们在测试集对MSE进行测量:
